Neural Networks And Fuzzy Control for Bulk Terminal Operating Management
From Proceeding of Summer Computer Simulation Conference,SCSC95, Ottawa, July 24-26 1994
Pietro Giribone, Agostino G.Bruzzone
THE COMPARISON BETWEEN SIMULATION AND PREDICTIONS
The decision support set was then tested on the simulation model, making it operate in an integrated mode. The statistical
analysis of the performances obtained showed the efficiency of the developed neural model.
Integrated use also indicated the efficiency of the proposed support compared with the automatic operating method already
implemented in the simulation model.
Despite the positive results obtained and the convenience of the approach compared to traditional methods, the final error
obtained in the predictive estimate of yard occupation supplied by the neural network still remains high (an error of about
10% of the estimated value).
However, since this involves a particularly complex chaotic system, it would be difficult to
obtain better results.
For this purpose, it was possible to verify the chaotic nature of the system by reconstructing the image of the relative
strange attractor. The time series produced by each simulation run is not predictable; therefore, it was decided to test this
phenomenon.
In effect, the capacity to predict the availability of material in the yard turns out to be a fundamental
operating management parameter.
This research team has already carried out various analyses relative to this type of problem, trying to classify the strange
attractors using numerical correlation techniques and then reprocessing the data to obtain a corresponding risk analysis.
However, it is very complex to obtain a classification of the system starting from data that can be measured directly on the
simulator in reasonable time periods.
It is for this very reason that it is thought that the use of neural models may be suitable for this type of application.
In this case, the yard quantities and their development at time t (corresponding to the flow of material) were considered as
input variables. Reference was made to the corresponding variables at time t+delta t as outputs.
Reference was made to previous studies in the sector relative to the possibility of applying these models to the study of
chaotic systems.
Network with two hidden layers were used with modified back-propagation algorithms (called temporal
back-propagation algorithms).
The results relative to the predictive capacity are illustrated in fig. 3.
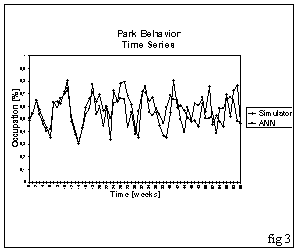
It can be seen that the expected development
compared to the real development, once a certain interval had elapsed, begins to diverge. The time error development is
reported in fig. 4.
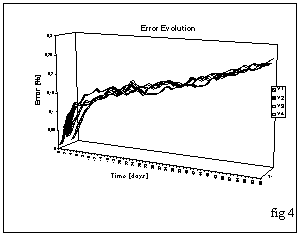
This curve provides an estimate of the operating time frame on which the model can operate as a
decision support.
However, comparing the attractors developed with the simulator with those produced by the neural network it is possible to
verify that qualitatively the behaviour of the system does seem to be effectively reproduced.